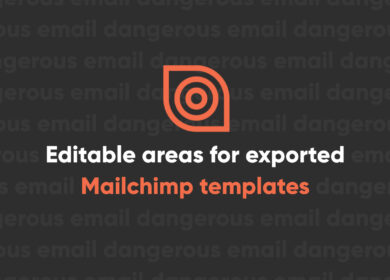
5 Ways You’re Doing A/B Testing Wrong
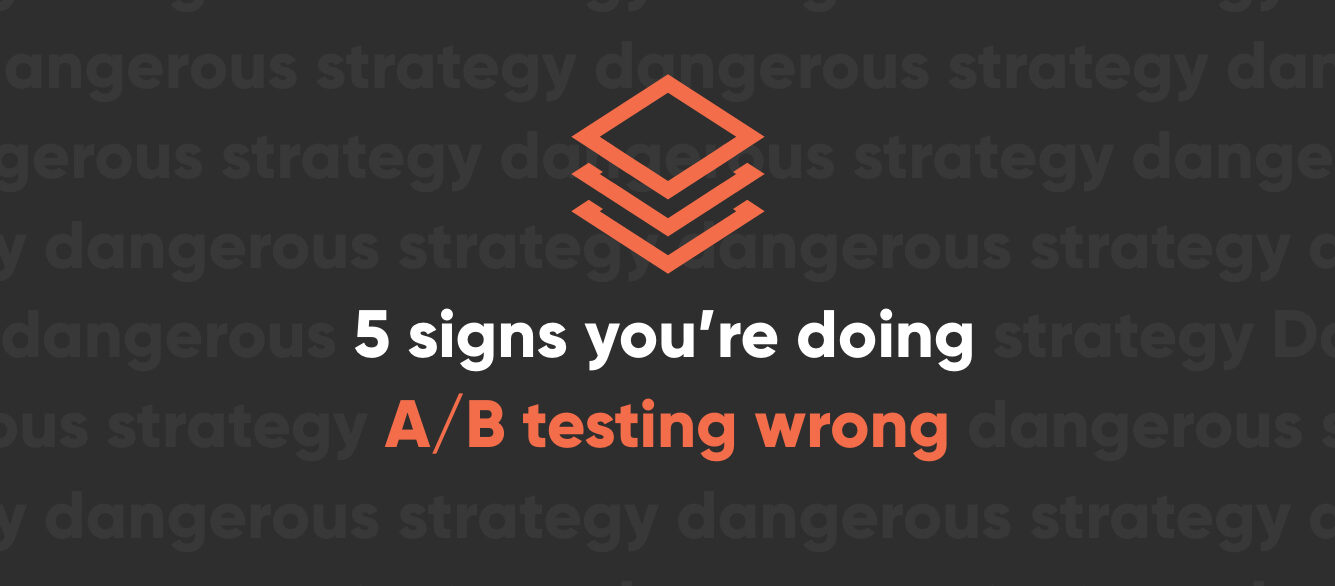
To get the most out of your website, you need to know what works. While following general best practices is a great way to start, the only true way to know what will work best for your website is by using data.
Cue the A/B test. Used properly, A/B testing is one of the most powerful things in a marketer’s toolbox. Unfortunately, many A/B tests are poorly conceived and result in misinformed decisions that don’t actually improve your conversions.
What Is A/B Testing?
In simple terms, an A/B test is a test of the effectiveness of different versions of an element. The basic idea is to see which option works better. Here’s how it works: you choose an element on your website, such as a "Buy Now" button. You create a variable, such as the color of the button. You randomly serve two different colors to your website visitors. You gather and analyze the data to determine which color drives more checkouts. When there is enough data, you can make an informed change, keep the element as it was, or run an additional test.
Seems simple enough, right? Well, you’d be surprise how often A/B tests are performed the wrong way. A bad A/B test can lead to a decision that isn’t in the best interest of your website. At best, these bad A/B tests are a waste of time. At worst, they can end up costing you a lot of money in the long run.
Here are 5 common A/B testing mistakes that can lead to bad business decisions.
Not Collecting Enough Data
Probably the most common mistake in A/B testing is failing to collect a significant amount of data. Statistically significant, that is.
Here’s what often happens with an A/B test. Someone will say, "Hey, let’s test a different contact form with fewer fields to see which gets more leads." Great! But then they set up the A/B test, wait a month, and declare a winner.
Look at the above example. Some people would be quick to say that Version 2 of this Quote Form is the clear winner because it had twice as many form submissions. But with only 66 total views and just 6 conversions, this data is far from conclusive.
Before you can declare a winner in an A/B test, you need to be sure you have a big enough sample size and enough conversion data. Luckily, there are many free tools available to help you. This calculator by AB Testguide is a pretty powerful one.
If you declare a winner too early, there’s a pretty good chance the results had little to do with the A/B test and more to do with random chance. This mistake can cause you to switch an element on your site to something that actually isn’t any better. In fact, something that performed better a single month might actually perform a lot worse over a longer period of time. Declaring a winner without enough data can make you into the loser.
Testing the Wrong Thing
There are tons of possibilities for A/B tests. Headlines, text copy, images, layouts, calls to action, form fields, colors, and much more. Basically, if something exists on your website, you can A/B test it. But, you don’t want to test just anything. An A/B test should be based on your goals and KPIs. Testing the size of the links in your footer probably isn’t going to lead to more conversions.
In many cases, an A/B test should be used when you notice an area of weakness on your site. If your checkout-to-order conversion rate is 99%, you don’t need to test a different checkout process. If your service pages are getting thousands of visits but you aren’t getting any quote requests, you probably need to test something. Testing your call to action might be one place to start.
But before you run that test, make sure you aren’t choosing a variable that isn’t related to the data you are actually trying to test. For example, if you are trying to increase the conversion rate of your contact form, changing the color of your call to action buttons might not be the right test. Changing the color of these buttons might affect the number of people who click-through to your contact form, but it may not have a direct impact on the number of people who complete your contact form. That doesn’t mean you shouldn’t test these things. You just need to have a clear understanding of where to start.
Testing Too Many Things with the Same Test
Another common error in A/B testing is trying to test too many things at the same time. Here’s a prime example:
You currently have a blue "Learn More" button at the bottom of your service page. You decide you want to test this because you aren’t getting many leads. Your test button is a red "Get a Free Quote" button in the sidebar of the page. You set up an A/B test to randomly serve one of these two buttons.
Here’s the potential problem with running a test like this: you have three variables and only one way to track it. How will you know if any improvement is because of the different color, different wording, or different position? Maybe a blue "Learn More" button in the sidebar would have generated even more leads than that red "Get a Free Quote" button.
This type of testing isn’t pointless though. After all, if your red "Get a Free Quote" button in the sidebar does produce more leads, then at least you’ve made a positive change for the website. However, you might have even better results if you focus on one variable at a time—or if you test more options. If you’re testing multiple elements at once, you need to have more than just two options.
Testing By Changing
I call this the lazy man’s A/B test. Instead of actually testing a variable, you simply make a change to your website, collect the data, and then compare it to previous data.
Let’s use the same call to action button example from before. Instead of randomly serving the blue "Learn More" button and the red "Get a Free Quote" button, you simply remove the "Learn More" button and put a "Get a Free Quote" button on the website instead. At the end of a month, you look at the data and say, "Well, there were more quote requests during May than there were in April, so this new button is much better than the old one."
There are many obvious problems here, including the amount of data you’re using, any seasonal trends, and whether or not the different button had any direct impact on quote requests. Maybe leaving the old button on the site would have resulted in the same number of quote requests as that new button. You can’t accurately compare what happened this month with one button to what happened last month with a different button.
That doesn’t mean you always have to run an A/B test before making a change to a website, but declaring an obvious winner without doing any testing is misguided in most cases.
Testing Two Things Simultaneously
This is the "choose your own adventure" style of A/B testing. Instead of randomly serving two options, you give your user both options at once. Then the user gets to pick which one he/she thinks is better. What could be more conclusive than this? You’re really putting it into the hands of the people!
An obviously bad is example of this is putting two versions of a contact form side by side on the same page. Which one will people fill out more? The form with more fields or the form with fewer fields? The answer is probably neither. Your users will be so confused by the double contact form that they’ll leave the site.
A less horrible example is having two different call to action buttons on the same page. You see which one gets clicked more, and that’s clearly the better one, right? Not so fast. In this case, you aren’t actually testing which is better. If you have a red call to action at the top of the page and a blue one at the bottom, seeing which one is clicked more doesn’t tell you which one is better. Rather, it tells you where people are clicking. In this case, if both of them are getting clicked, you probably want to keep both of them.
Conclusion
A/B testing is a vital part of every successful website. If you never test anything, then you’ll never know what you could be doing better. But before you run an A/B test, make sure you have a plan. Know what you need to test, how you are going to test it, how you are going to track it, how much data you need, and what you’re going to do with that data. Ultimately, an A/B testing error probably won’t ruin your business, but it will be a big waste of your time.

Nate Tower
Nate Tower is the President of Perrill and has over 12 years of marketing and sales experience. During his career in digital marketing, Nate has demonstrated exceptional skills in strategic planning, creative ideation and execution. Nate's academic background includes a B.A. with a double major in English Language and Literature, Secondary Education, and a minor in Creative Writing from Washington University. He further expanded his expertise by completing the MBA Essentials program at Carlson Executive Education, University of Minnesota.
Nate holds multiple certifications from HubSpot and Google including Sales Hub Enterprise Implementation, Google Analytics for Power Users and Google Analytics 4. His unique blend of creative and analytical skills positions him as a leader in both the marketing and creative worlds. This, coupled with his passion for learning and educating, lends him the ability to make the complex accessible and the perplexing clear.
Author
Nate Tower
Categories
Date
Explore with AI
Join Our Newsletter
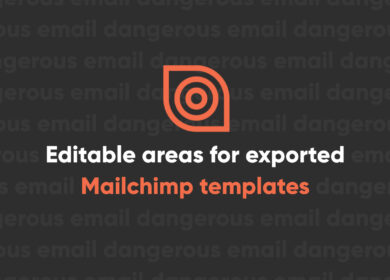
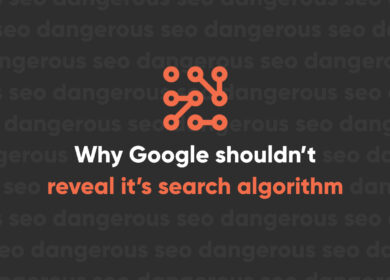
Why Google Shouldn’t Reveal Its Search Algorithm

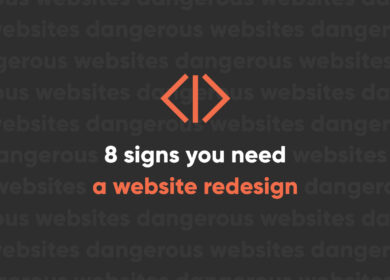
8 Signs You Need a Website Redesign
